Overview:
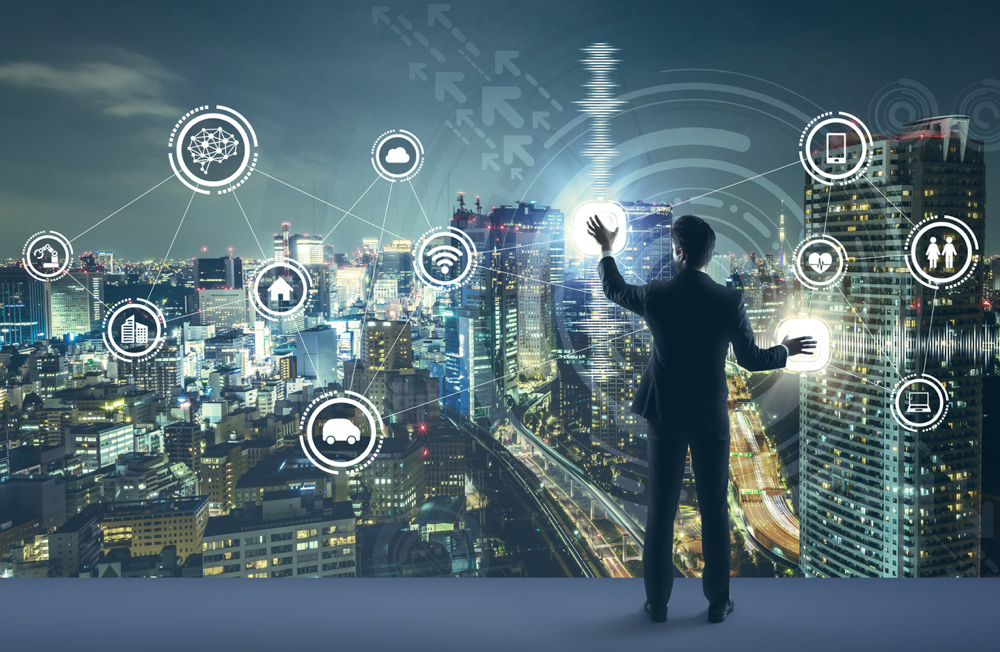
Energy Utilization Forecast:
Request Guaging: RL algorithms foresee future energy needs by examining authentic information, empowering more proficient energy dispersion and diminished wastage.
Load Moving: By anticipating high and low utilization periods, algorithms can move trivial energy utilization to off busy times, decreasing expenses and stress on the lattice.
Automated Control Frameworks:
Shrewd Indoor regulators: RL algorithms upgrade warming and cooling plans in light of inhabitance examples and weather conditions gauges, maintain with solace while limiting energy use.
Lighting Control: Automated lighting frameworks use RL to change splendor and on/off plans as indicated by inhabitance and regular light accessibility.
Combination with Sustainable Power Sources:
Sun oriented Power The board: RL algorithms deal with the utilization of solar energy, enhancing the harmony between energy capacity and direct use to amplify proficiency.
Energy Capacity Advancement: These algorithms decide the best times to charge and release home batteries, ensuring sustainable power is used successfully.
Ongoing Input and Proposals:
Client Commitment: Mortgage holders get continuous criticism on their energy utilization designs and customized proposals to upgrade proficiency.
Conduct Pokes: RL algorithms can recommend little conduct changes that affect the total outcome of huge energy reserve funds.
All in all, reinforcement learning algorithms upgrade energy effectiveness in smart homes by gaining from and adjusting to occupants' ways of behaving, enhancing the utilization of sustainable power, overseeing apparatus plans, and giving ongoing criticism. This comprehensive methodology ensures decreased energy utilization, lower costs, and further developed manageability.
Read more: How can reinforcement learning optimize traffic patterns in smart cities