Overview:
Deep learning is a subset of contraption acquiring information that has practical experience in tutoring engineered brain networks with two or three layers to look at complex examples and portrayals from information. While every profound acquisition of information on and traditional device dominating aim to permit PCs to analyze realities and settle on forecasts or decisions, they fluctuate in various key parts:
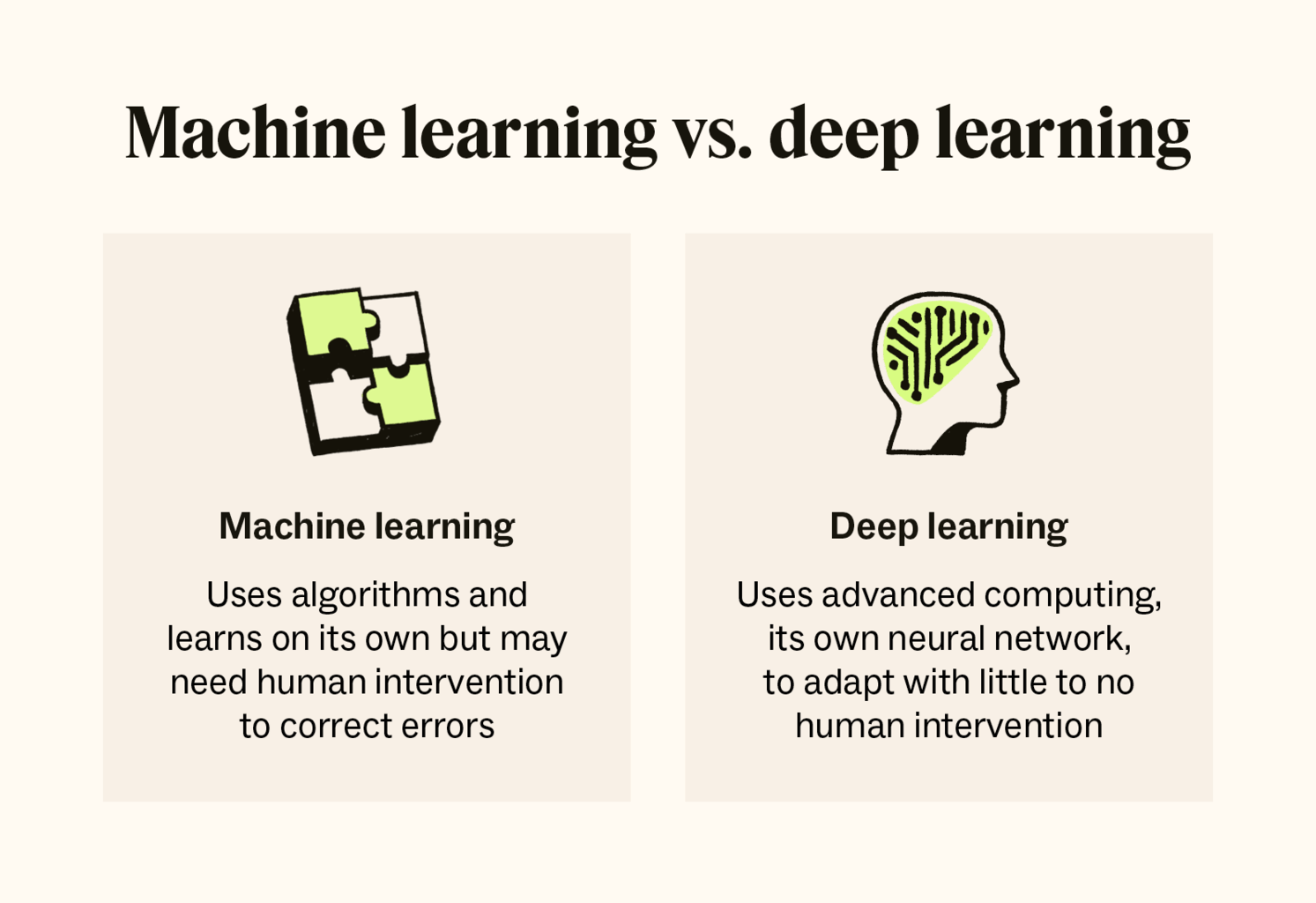
- Portrayal of Information: In customary AI, capability designing is for the most part required, where specialists physically pick and plan abilities from uncooked realities to address input for the concentrating on set of rules. In appraisal, profound concentration naturally gains material highlights from uncooked data through progressive layers of deliberation, pushing off the need for manual trademark designing.
- Design Intricacy: Profound learning styles, mostly profound brain organizations, envelop different layers of interconnected hubs (neurons), permitting them to concentrate on tricky styles and connections in information. These styles can hold onto complex nonlinear connections, making them suitable for undertakings with colossal, unstructured datasets. Customary device learning calculations, which incorporate choice trees or help vector machines, frequently have less difficult structures and may be fighting to address high-layered data.
- Preparing Interaction: Profound acquiring information on designs requires sizable measures of arranged insights for training and rely on streamlining methodologies like stochastic angle drop to iteratively alter the loads of associations among neurons. Preparing profound brain organizations can be computationally broad and tedious, requiring viable equipment that incorporates GPUs or TPUs. In assessment, customary AI calculations may also require significantly less measurements and computational resources for preparing.
- Execution and Adaptability: Profound dominating models have approved outstanding execution in assignments along with picture ubiquity, normal language handling, and discourse notoriety, beating conventional machines concentrating on strategies in bunches of cases. In any case, profound concentrating on models may likewise be more noteworthy helpless to overfitting, for the most part with obliged preparing realities. Furthermore, sending and scaling profoundly concentrating on styles might be trying because of their computational necessities and intricacy.
Generally speaking, while both deep learning to be aware and traditional device acquiring information on have their assets and shortcomings, profound acquiring information on succeeds in overseeing confounded, high-layered measurements and has achieved extensive upgrades in different fields, which incorporates pc vision, discourse notoriety, and autonomous designs.
Read more: What are some real-world applications of machine learning